New App Enables Conservationists to Quickly Mine Research for Key Insights
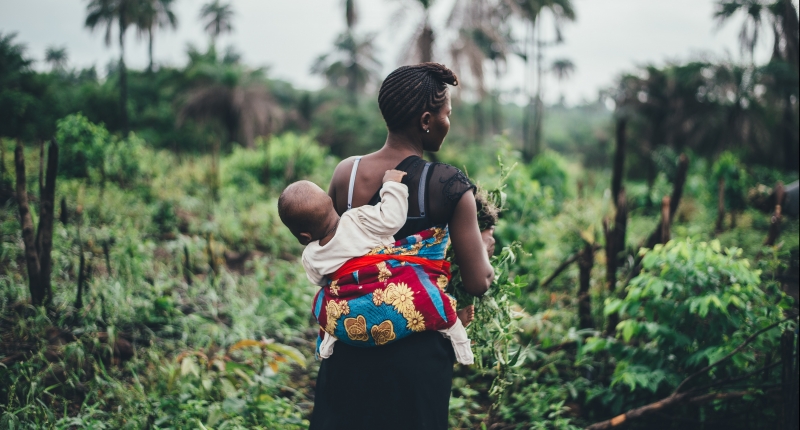
Determining the best course of action for protecting an ecosystem and the human livelihoods dependent on it is no quick and easy process, despite the urgency often felt around it. It can take months, even years of sifting through piles of studies to track down the evidence needed to make the right decision – until now.
Researchers from the Science for Nature and People Partnership (SNAPP), in partnership with Conservation International and DataKind, recently launched Colandr, an open-access machine learning application that allows for faster sifting and winnowing of scientific data to help conservation practitioners and policymakers find the evidence they need to make science-based decisions more quickly than ever before.
“In the context of the global challenges we are facing, we can’t be waiting years for people to comb through the information to understand what is the most effective action to take,” said project lead Samantha Cheng, a postdoctoral researcher with SNAPP Working Group on Evidence-Based Conservation. “Colandr not only has the potential to help us find this information faster, but is completely open access, allowing anyone to use it for free.”
Created by a DataKind team of pro-bono developers and data scientists, Colandr helps users quickly locate the proverbial needle(s) in the information haystack. Through machine learning, the application has the ability to learn on its own and keep pace with the volume and speed of the ever-expanding body of knowledge, while also allowing researchers to search rapidly without losing the precision and accuracy of a real, live person. As users upload, sort and select conservation literature, the app simultaneously learns from users’ input and fetches what is most relevant to them.
For example, researchers from Conservation International used Colandr to pare down evidence on synergies, tradeoffs and equity in marine conservation to help them more quickly determine the state of the science, information that will guide their efforts moving forward.
“New technologies such as machine learning and text-mining have tremendous potential to help us tackle the ‘big data’ problem facing science today,” explained Cheng, whose own year-and-a-half long hunt through 35,000 papers for a recent project on links between conservation and human well-being motivated Colandr’s creation.
Colandr is both open access and open source, making it not only free for anyone to use, but also transparent so other developers can examine and reuse the code or enhance the tool’s capacity to serve the conservation community.
“Scientists want to feel comfortable with the decisions they are making, not a black box making decisions for them,” said Cheng. “Taking an open source approach is especially important for conservation, as both time and resources for decision-making are limited – open source and open access methods can help push the entire community along together, avoiding the risk of individually reinventing the wheel.”
A community webpage built into the app provides access to the underlying code, as well as opportunities for user interaction and conversation.
"It's inspiring to see data science and machine-learning solutions being put into practice like this, tackling a problem that has stymied the research community for years, and to know the potential impact this can have for conservation community as well as other sectors," said collaborator JeanCarlo Bonilla, data science manager for the project and the director of insights and impact at the nonprofit DataKind.